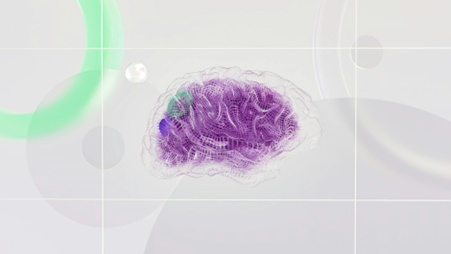
AI and Machine Learning
Have you ever wondered how your smartphone recognizes your face, or how Netflix seems to know exactly what you want to watch next? Welcome to the fascinating world of AI and Machine Learning – technologies that are reshaping our daily lives in ways we might not even realize! 🤖💡
From healthcare to finance, entertainment to transportation, AI and ML are revolutionizing industries at breakneck speed. But with this rapid advancement comes a host of questions: How do these technologies really work? What are their implications for our society? And most importantly, how can you harness their power? In this blog post, we’ll demystify AI and Machine Learning, explore their impact across various sectors, and delve into the ethical considerations that come with such powerful tools. Whether you’re a tech enthusiast or simply curious about the future, join us as we unravel the complexities of AI and ML, and discover how you can get started in this exciting field. Let’s embark on a journey through the present and future of artificial intelligence and machine learning!
10 Ways AI is Transforming Everyday Life in 2024
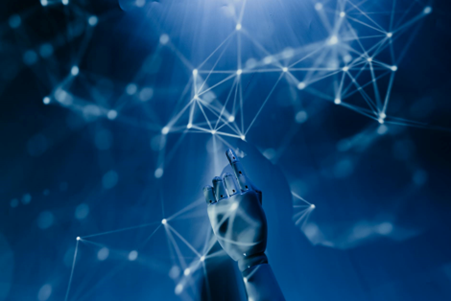
Demystifying AI and Machine Learning
As we dive into the fascinating world of Artificial Intelligence (AI) and Machine Learning (ML), it’s crucial to understand these technologies that are reshaping our world. Let’s explore the key differences between AI and ML, their real-world applications, and how they work together to create powerful solutions.
A. Key differences between AI and Machine Learning
While AI and ML are often used interchangeably, they are distinct concepts with unique characteristics. Understanding these differences is essential for grasping their individual roles and potential.
1. Definition and Scope
Machine Learning (ML): ML is a subset of AI that focuses on developing algorithms and statistical models that enable computer systems to improve their performance on a specific task through experience, without being explicitly programmed.
Artificial Intelligence (AI): AI is a broad field of computer science that aims to create intelligent machines capable of performing tasks that typically require human intelligence. These tasks include reasoning, problem-solving, learning, perception, and natural language processing.
2. Approach and Methodology
AI: AI encompasses a wide range of approaches, including rule-based systems, expert systems, and machine learning. It can involve both symbolic (logic-based) and sub-symbolic (data-driven) methods.
ML: ML relies primarily on data-driven approaches, using statistical techniques to enable systems to learn patterns and make predictions or decisions based on input data.
3. Human Intervention
AI: Some AI systems require extensive human intervention and programming to define rules and knowledge bases. These are often referred to as “symbolic AI” or “good old-fashioned AI” (GOFAI).
ML: ML systems are designed to learn from data with minimal human intervention. Once trained, they can make predictions or decisions with little to no human input.
4. Adaptability
AI: Traditional AI systems may struggle to adapt to new situations without human intervention or reprogramming.
ML: ML algorithms are designed to improve and adapt their performance as they are exposed to more data, making them more flexible in handling new situations.
5. Problem-Solving Approach
AI: AI systems can be designed to tackle a wide range of problems, from narrow, specific tasks to more general, human-like reasoning.
ML: ML is primarily focused on finding patterns in data and making predictions or decisions based on those patterns.
6. Dependency on Data
AI: While some AI systems rely heavily on data, others can be built using expert knowledge and rules without requiring large datasets.
ML: ML algorithms are heavily dependent on large, high-quality datasets for training and improving their performance.
To better illustrate these differences, let’s look at a comparison table:
Aspect | Artificial Intelligence | Machine Learning |
Definition | Creating intelligent machines capable of human-like tasks | Developing algorithms that improve through experience |
Scope | Broad field encompassing various approaches | Subset of AI focused on data-driven learning |
Goal | Simulate human intelligence | Identify patterns and make predictions from data |
Approaches | Rule-based, expert systems, machine learning, etc. | Supervised, unsupervised, reinforcement learning |
Human Intervention | Can require extensive programming and knowledge input | Minimal intervention after initial setup and training |
Adaptability | May struggle with new situations without reprogramming | Designed to improve and adapt with more data |
Data Dependency | Varies depending on the approach | Heavily dependent on large, quality datasets |
Problem-Solving | Can tackle a wide range of problems | Focused on pattern recognition and prediction |
Understanding these key differences helps in appreciating the unique strengths and applications of both AI and ML. Now, let’s explore some real-world applications that showcase the power of these technologies.
B. Real-world applications
AI and ML have found their way into numerous industries and aspects of our daily lives. Their applications range from enhancing user experiences to solving complex scientific problems. Let’s examine some of the most impactful and innovative uses of AI and ML across various sectors.
1. Healthcare
The healthcare industry has seen significant advancements through the integration of AI and ML technologies. These applications are improving patient care, diagnostics, and treatment planning.
- Medical Imaging and Diagnostics: ML algorithms, particularly deep learning neural networks, have shown remarkable accuracy in analyzing medical images such as X-rays, MRIs, and CT scans. These systems can detect anomalies and assist radiologists in identifying potential issues like tumors, fractures, or other abnormalities.
Example: Google’s DeepMind has developed an AI system that can detect over 50 eye diseases with accuracy comparable to expert ophthalmologists.
- Personalized Treatment Plans: AI systems can analyze vast amounts of patient data, including genetic information, medical history, and lifestyle factors, to suggest personalized treatment plans. This approach, known as precision medicine, helps healthcare providers tailor treatments to individual patients for better outcomes.
- Drug Discovery: ML algorithms are accelerating the drug discovery process by predicting how different chemical compounds will interact with biological targets. This can significantly reduce the time and cost associated with developing new medications.
Example: Atomwise used AI to identify two drugs that could potentially reduce Ebola infectivity, accomplishing in less than a day what would have traditionally taken months or years.
- Predictive Healthcare: ML models can analyze patient data to predict potential health risks and recommend preventive measures. This proactive approach can help in early detection of diseases and improve overall patient outcomes.
2. Finance and Banking
The financial sector has embraced AI and ML to enhance security, improve customer service, and optimize investment strategies.
- Fraud Detection: ML algorithms can analyze transaction patterns in real-time to identify potentially fraudulent activities. These systems can learn from new data and adapt to evolving fraud techniques, providing robust protection for financial institutions and their customers.
- Algorithmic Trading: AI-powered trading systems can analyze market trends, news, and other relevant data to make rapid trading decisions. These systems can execute trades at speeds and frequencies impossible for human traders.
- Credit Scoring: ML models can assess creditworthiness more accurately by considering a wider range of factors than traditional methods. This can lead to fairer lending practices and better risk assessment for financial institutions.
- Customer Service: AI-powered chatbots and virtual assistants are revolutionizing customer service in the banking sector. These systems can handle a wide range of customer queries, from account inquiries to complex financial advice, 24/7.
Example: Bank of America’s virtual assistant, Erica, has served over 10 million users, handling tasks from balance inquiries to bill payments and credit report assistance.
3. Retail and E-commerce
AI and ML are transforming the retail landscape, both online and in physical stores, by personalizing shopping experiences and optimizing operations.
- Recommendation Systems: ML algorithms analyze user behavior, purchase history, and product attributes to provide personalized product recommendations. These systems significantly improve customer engagement and increase sales.
Example: Amazon’s recommendation engine is responsible for 35% of the company’s total sales, showcasing the power of ML in driving e-commerce revenue.
- Inventory Management: AI systems can predict demand patterns, optimize stock levels, and manage supply chain logistics more efficiently. This leads to reduced costs and improved product availability.
- Price Optimization: ML algorithms can analyze market trends, competitor pricing, and demand patterns to suggest optimal pricing strategies in real-time, maximizing profits while remaining competitive.
- Visual Search: AI-powered visual search allows customers to find products by uploading images, enhancing the shopping experience and making product discovery more intuitive.
Example: Pinterest’s visual search feature, Lens, uses computer vision to identify objects in images and suggest similar products, enhancing user engagement and driving sales for partnered retailers.
4. Transportation and Automotive
The transportation industry is being revolutionized by AI and ML, with applications ranging from autonomous vehicles to traffic management systems.
- Autonomous Vehicles: Self-driving cars use a combination of AI technologies, including computer vision, sensor fusion, and decision-making algorithms, to navigate roads safely without human intervention.
Example: Waymo, Google’s self-driving car project, has driven over 20 million miles autonomously, showcasing the potential of AI in transforming personal transportation.
- Traffic Management: AI systems can analyze real-time traffic data from various sources to optimize traffic flow, reduce congestion, and improve overall transportation efficiency in urban areas.
- Predictive Maintenance: ML algorithms can analyze sensor data from vehicles to predict maintenance needs, reducing downtime and improving safety.
- Ride-Sharing Optimization: Companies like Uber and Lyft use ML algorithms to optimize route planning, pricing, and driver-passenger matching, improving efficiency and user experience.
5. Education
AI and ML are enhancing educational experiences and outcomes through personalized learning and administrative support.
- Adaptive Learning Platforms: ML algorithms can analyze student performance and learning patterns to create personalized learning paths, adapting content difficulty and pacing to individual needs.
Example: Carnegie Learning’s MATHia platform uses AI to provide personalized math instruction, adjusting in real-time based on student responses and learning pace.
- Automated Grading: AI systems can grade multiple-choice questions and even evaluate written responses, freeing up teachers’ time for more impactful activities.
- Student Performance Prediction: ML models can analyze various factors to predict student performance and identify those at risk of falling behind, allowing for timely interventions.
- Intelligent Tutoring Systems: AI-powered tutoring systems can provide personalized assistance to students, answering questions and guiding them through problem-solving processes.
6. Manufacturing and Industry
AI and ML are driving the fourth industrial revolution, often referred to as Industry 4.0, by optimizing production processes and enabling predictive maintenance.
- Quality Control: Computer vision systems powered by deep learning can detect product defects with high accuracy, improving quality control processes.
- Predictive Maintenance: ML algorithms can analyze sensor data from machinery to predict when maintenance is needed, reducing downtime and extending equipment lifespan.
Example: Siemens uses AI-powered predictive maintenance in its gas turbines, reducing inspection time by 75% and significantly improving reliability.
- Supply Chain Optimization: AI systems can analyze complex supply chain data to optimize logistics, reduce costs, and improve efficiency.
- Robotics: AI-powered robots are becoming increasingly sophisticated, capable of performing complex tasks in manufacturing environments with high precision and adaptability.
7. Energy and Utilities
The energy sector is leveraging AI and ML to improve efficiency, reduce costs, and transition to more sustainable practices.
- Smart Grids: AI systems can optimize energy distribution in power grids, balancing supply and demand in real-time and integrating renewable energy sources more efficiently.
- Energy Consumption Prediction: ML models can analyze historical data and various factors to predict energy consumption patterns, helping utilities prepare for demand spikes.
- Fault Detection: AI algorithms can analyze sensor data from power infrastructure to detect potential faults before they cause outages, improving grid reliability.
Example: Google’s DeepMind AI has been used to optimize cooling systems in data centers, reducing energy consumption for cooling by up to 40%.
8. Agriculture
AI and ML are revolutionizing agriculture through precision farming techniques and crop management.
- Crop and Soil Monitoring: Computer vision and ML algorithms can analyze satellite and drone imagery to assess crop health, predict yields, and detect issues like pest infestations or nutrient deficiencies.
- Precision Agriculture: AI-powered systems can optimize irrigation, fertilization, and pest control based on real-time data and predictive models, improving yields and reducing resource use.
Example: The FarmBeats project by Microsoft uses AI and IoT sensors to provide data-driven insights to farmers, helping them make more informed decisions about crop management.
- Livestock Management: ML algorithms can monitor animal health, optimize feeding schedules, and even predict disease outbreaks in livestock.
9. Environmental Protection and Climate Change
AI and ML are playing crucial roles in understanding and addressing environmental challenges.
- Climate Modeling: ML models can analyze vast amounts of climate data to improve climate change predictions and help in developing mitigation strategies.
- Wildlife Conservation: AI-powered systems can analyze camera trap images and acoustic data to monitor wildlife populations and detect poaching activities.
Example: The PAWS (Protection Assistant for Wildlife Security) project uses AI to predict poaching threats and optimize ranger patrols in wildlife reserves.
- Disaster Prediction and Response: ML algorithms can analyze satellite imagery and weather data to predict natural disasters like floods or wildfires, enabling better preparedness and response.
10. Entertainment and Media
The entertainment industry is leveraging AI and ML to enhance content creation, personalization, and user experiences.
- Content Recommendation: Streaming platforms like Netflix and Spotify use sophisticated ML algorithms to recommend movies, shows, and music based on user preferences and behavior.
- Content Creation: AI is being used to assist in various aspects of content creation, from generating music to writing scripts and even creating visual effects.
Example: The short film “Sunspring” was entirely written by an AI system trained on science fiction screenplays, showcasing the potential of AI in creative processes.
- Gaming: AI is used in video games to create more realistic non-player characters (NPCs), generate dynamic game environments, and even adapt game difficulty based on player performance.
These real-world applications demonstrate the transformative power of AI and ML across various industries. As these technologies continue to evolve, we can expect even more innovative and impactful applications in the future. Now, let’s explore how AI and ML work together to create these powerful solutions.The entertainment industry is leveraging AI and ML to enhance content creation, personalization, and user experiences.
- Content Recommendation: Streaming platforms like Netflix and Spotify use sophisticated ML algorithms to recommend movies, shows, and music based on user preferences and behavior.
- Content Creation: AI is being used to assist in various aspects of content creation, from generating music to writing scripts and even creating visual effects.
Example: The short film “Sunspring” was entirely written by an AI system trained on science fiction screenplays, showcasing the potential of AI in creative processes.
- Gaming: AI is used in video games to create more realistic non-player characters (NPCs), generate dynamic game environments, and even adapt game difficulty based on player performance.
These real-world applications demonstrate the transformative power of AI and ML across various industries. As these technologies continue to evolve, we can expect even more innovative and impactful applications in the future. Now, let’s explore how AI and ML work together to create these powerful solutions.
How AI and ML work together
AI and ML are deeply intertwined, with ML serving as a key approach within the broader field of AI. Understanding how these technologies complement each other is crucial for grasping their full potential and limitations. Let’s explore the synergy between AI and ML and how they collaborate to create powerful solutions.
1. The AI-ML Relationship
AI and ML have a hierarchical relationship, with ML being a subset of AI. Here’s how they interact:
- AI as the Overarching Goal: AI represents the broader vision of creating intelligent machines capable of performing tasks that typically require human intelligence. This encompasses various approaches, including but not limited to ML.
- ML as a Key Approach: Machine Learning is one of the most successful and widely used approaches to achieving AI. It focuses on enabling systems to learn from data and improve their performance over time.
- Complementary Strengths: While ML excels at pattern recognition and prediction based on large datasets, other AI approaches can handle tasks that require reasoning, planning, and knowledge representation.
2. The ML Pipeline in AI Systems
Machine Learning often forms the core of many AI systems, following a general pipeline:
- Data Collection and Preparation: Gathering relevant data and preprocessing it for use in ML algorithms.
- Feature Engineering: Selecting or creating the most relevant features from the data for the task at hand.
- Model Selection and Training: Choosing appropriate ML algorithms and training them on the prepared data.
- Model Evaluation and Tuning: Assessing the model’s performance and adjusting parameters to improve results.
- Deployment and Integration: Incorporating the trained ML model into a larger AI system or application.
This ML pipeline is often embedded within a broader AI framework that may include other components like natural language processing, computer vision, or expert systems.
3. Hybrid AI Systems
Many modern AI applications combine ML with other AI techniques to create more robust and versatile systems. Some examples include:
- Combining ML with Knowledge Bases: Integrating ML models with structured knowledge bases can enhance the system’s ability to reason and make decisions based on both learned patterns and explicit rules.
Example: In healthcare diagnosis systems, ML models can be combined with medical knowledge bases to provide more accurate and explainable diagnoses.
- Ensemble Methods: Using multiple ML models in conjunction, each specialized for different aspects of a problem, can lead to more accurate and reliable predictions.
- Reinforcement Learning with Planning: Combining reinforcement learning (an ML technique) with AI planning algorithms can create systems that learn from experience while also reasoning about long-term consequences of actions.
Example: DeepMind’s AlphaGo, which defeated world champions in the game of Go, combines deep learning (an ML technique) with tree search algorithms (a traditional AI approach) to achieve superhuman performance.
4. AI-Driven ML Optimization
AI techniques are increasingly being used to optimize ML processes themselves:
- Automated Machine Learning (AutoML): AI systems can automate the process of selecting and tuning ML models, making ML more accessible and efficient.
Example: Google’s Cloud AutoML allows users to create high-quality custom ML models with minimal ML expertise.
- Neural Architecture Search: AI algorithms can design optimal neural network architectures for specific tasks, potentially outperforming human-designed models.
- Intelligent Data Preprocessing: AI systems can assist in data cleaning, feature selection, and data augmentation, improving the quality of input for ML models.
5. Continuous Learning and Adaptation
The integration of AI and ML enables systems to continuously learn and adapt:
- Online Learning: ML models can be updated in real-time as new data becomes available, allowing AI systems to adapt to changing environments or user behaviors.
- Transfer Learning: Knowledge gained by ML models in one domain can be transferred to related tasks, enabling faster learning and better performance in new situations.
Example: In natural language processing, models like BERT are pre-trained on vast amounts of text data and then fine-tuned for specific tasks like sentiment analysis or question answering.
6. Explainable AI (XAI)
As AI systems become more complex, there’s a growing need for explainability:
- Interpretable ML Models: Developing ML models that can provide explanations for their decisions is crucial for building trust and understanding in AI systems.
- AI-Powered Explanations: AI techniques can be used to generate human-understandable explanations for complex ML model decisions.
Example: In financial services, XAI techniques are used to explain credit scoring decisions, ensuring transparency and compliance with regulations.
7. Edge AI

The Impact of AI and ML on Industries
As we delve deeper into the world of AI and machine learning, it’s crucial to understand how these technologies are revolutionizing various industries. From retail to healthcare, AI and ML are transforming the way businesses operate, enhancing efficiency, and creating new opportunities for growth and innovation.
A. Retail Personalization
Artificial intelligence and machine learning algorithms have significantly transformed the retail industry, offering unprecedented levels of personalization and customer engagement. This revolution has reshaped the shopping experience, both online and in physical stores, creating a more tailored and efficient environment for consumers.
1. Enhanced Customer Profiling
AI-powered systems can analyze vast amounts of customer data, including browsing history, purchase patterns, and demographic information, to create detailed customer profiles. These profiles enable retailers to understand individual preferences, anticipate needs, and offer personalized product recommendations.
- Behavioral Analysis: AI algorithms can track and analyze customer behavior across various touchpoints, including websites, mobile apps, and in-store interactions.
- Predictive Modeling: Machine learning models can predict future purchasing behavior based on historical data and current trends.
- Segmentation: AI can automatically segment customers into distinct groups based on shared characteristics, allowing for more targeted marketing strategies.
2. Personalized Product Recommendations
One of the most visible applications of AI in retail is personalized product recommendations. These systems use complex algorithms to suggest items that are most likely to appeal to individual customers, increasing the likelihood of purchases and improving customer satisfaction.
- Collaborative Filtering: This technique recommends products based on the preferences of similar customers.
- Content-Based Filtering: This approach suggests items similar to those a customer has shown interest in previously.
- Hybrid Systems: Many retailers use a combination of both methods to provide more accurate recommendations.
3. Dynamic Pricing Strategies
AI and ML enable retailers to implement sophisticated dynamic pricing strategies, adjusting prices in real-time based on various factors such as demand, competitor pricing, and individual customer willingness to pay.
- Demand Forecasting: ML algorithms can predict demand fluctuations, allowing retailers to optimize pricing accordingly.
- Competitor Analysis: AI-powered tools can monitor competitor prices and automatically adjust pricing to remain competitive.
- Personalized Discounts: Retailers can offer individualized discounts based on a customer’s profile and purchase history.
4. Chatbots and Virtual Assistants
AI-powered chatbots and virtual assistants have become increasingly common in retail, providing 24/7 customer support and personalized shopping assistance.
- Natural Language Processing: Advanced NLP allows chatbots to understand and respond to customer queries in a more human-like manner.
- Product Recommendations: Virtual assistants can suggest products based on customer preferences and previous interactions.
- Order Tracking: Chatbots can provide real-time updates on order status and shipping information.
5. Visual Search and Augmented Reality
Innovative AI technologies like visual search and augmented reality (AR) are enhancing the online shopping experience, making it more interactive and engaging.
- Image Recognition: Customers can search for products by uploading images, with AI algorithms identifying similar items in the retailer’s inventory.
- Virtual Try-On: AR technology allows customers to virtually try on clothing, accessories, or makeup before making a purchase.
- In-Store Navigation: AR-powered apps can guide customers through physical stores, providing personalized product information and offers.
6. Inventory Management and Supply Chain Optimization
AI and ML are revolutionizing inventory management and supply chain operations, leading to improved efficiency and reduced costs.
- Demand Forecasting: ML algorithms can predict future demand with greater accuracy, helping retailers optimize their inventory levels.
- Automated Replenishment: AI-powered systems can automatically reorder stock when inventory levels fall below a certain threshold.
- Route Optimization: ML algorithms can optimize delivery routes, reducing transportation costs and improving delivery times.
7. Fraud Detection and Prevention
AI and ML play a crucial role in detecting and preventing fraud in retail transactions, protecting both retailers and customers.
- Anomaly Detection: ML algorithms can identify unusual patterns in transaction data that may indicate fraudulent activity.
- Real-Time Monitoring: AI systems can monitor transactions in real-time, flagging suspicious activities for immediate review.
- Adaptive Learning: ML models can continuously learn from new data, improving their ability to detect evolving fraud tactics.
8. Personalized Marketing Campaigns
AI enables retailers to create highly targeted marketing campaigns, improving engagement rates and return on investment.
- Content Personalization: AI can tailor marketing content to individual customers based on their preferences and behavior.
- Optimal Timing: ML algorithms can determine the best times to send marketing messages to maximize engagement.
- Cross-Channel Optimization: AI can coordinate marketing efforts across multiple channels for a cohesive customer experience.
9. In-Store Analytics
For brick-and-mortar retailers, AI and ML provide valuable insights into in-store customer behavior and operations.
- Foot Traffic Analysis: Computer vision and ML can analyze customer movement patterns within stores.
- Heat Mapping: AI can create heat maps showing which areas of a store receive the most attention from customers.
- Shelf Management: ML algorithms can optimize product placement on shelves based on customer behavior and sales data.
10. Voice Commerce
As voice-activated devices become more prevalent, AI-powered voice commerce is emerging as a new frontier in retail personalization.
- Voice Recognition: Advanced AI algorithms can accurately interpret and respond to voice commands.
- Personalized Recommendations: Voice assistants can provide personalized product suggestions based on a customer’s purchase history and preferences.
- Seamless Ordering: AI enables customers to place orders using voice commands, streamlining the purchasing process.
Aspect of Retail Personalization | AI/ML Application | Benefits |
Customer Profiling | Data analysis, predictive modeling | More accurate targeting, improved customer understanding |
Product Recommendations | Collaborative and content-based filtering | Increased sales, enhanced customer satisfaction |
Dynamic Pricing | Demand forecasting, competitor analysis | Optimized pricing, increased revenue |
Customer Support | Chatbots, virtual assistants | 24/7 support, reduced customer service costs |
Visual Search and AR | Image recognition, AR technology | Enhanced online shopping experience, reduced returns |
Inventory Management | Demand forecasting, automated replenishment | More Control Over Inventory Management |
Fraud Detection | Anomaly detection, real-time monitoring | Reduced fraud losses, increased customer trust |
Marketing Campaigns | Content personalization, optimal timing | Improved engagement rates, higher ROI |
In-Store Analytics | Foot traffic analysis, heat Voice | Optimized store layouts, improved customer experience |
Voice Commerce | Voice recognition, personalized recommendations | New sales channel, enhanced convenience |
The impact of AI and ML on retail personalization has been transformative, offering retailers powerful tools to understand and serve their customers better. As these technologies continue to evolve, we can expect even more innovative applications that will further enhance the shopping experience and drive retail growth.
B. Manufacturing Efficiency
The manufacturing industry has been greatly impacted by the integration of artificial intelligence and machine learning technologies. These advanced systems are revolutionizing production processes, improving efficiency, reducing costs, and enhancing product quality. Let’s explore the various ways AI and ML are transforming manufacturing efficiency.
1. Predictive Maintenance
One of the most significant applications of AI and ML in manufacturing is predictive maintenance. This approach uses advanced algorithms to predict when equipment is likely to fail, allowing for proactive maintenance and minimizing unexpected downtime.
- Sensor Data Analysis: ML algorithms analyze data from sensors placed on manufacturing equipment to detect anomalies and predict potential failures.
- Historical Data Integration: AI systems can integrate historical maintenance data with real-time sensor information for more accurate predictions.
- Maintenance Scheduling: Based on predictive analytics, AI can optimize maintenance schedules, reducing unnecessary maintenance while preventing unexpected breakdowns.
2. Quality Control and Defect Detection
AI-powered computer vision systems are revolutionizing quality control processes, offering faster and more accurate defect detection than traditional methods.
- Image Recognition: Advanced AI algorithms can analyze images or video feeds to detect defects in products on the production line.
- Real-Time Monitoring: ML systems can continuously monitor production processes, identifying quality issues as they occur.
- Adaptive Learning: AI quality control systems can learn from new data, continuously improving their defect detection capabilities.
3. Supply Chain Optimization
AI and ML are transforming supply chain management, enabling manufacturers to optimize their operations from raw material procurement to final product delivery.
- Demand Forecasting: ML algorithms can analyze historical data, market trends, and external factors to predict future demand more accurately.
- Inventory Optimization: AI can optimize inventory levels, reducing carrying costs while ensuring sufficient stock to meet demand.
- Supplier Performance Analysis: ML can analyze supplier performance data to identify the most reliable and cost-effective suppliers.
4. Process Optimization
AI and ML technologies are being used to optimize manufacturing processes, improving efficiency and reducing waste.
- Production Line Optimization: ML algorithms can analyze production data to identify bottlenecks and suggest improvements.
- Energy Efficiency: AI systems can optimize energy consumption in manufacturing facilities, reducing costs and environmental impact.
- Material Usage Optimization: ML can help optimize the use of raw materials, reducing waste and improving cost-efficiency.
5. Robotic Process Automation (RPA)
The integration of AI with robotics is leading to more intelligent and flexible automation solutions in manufacturing.
- Collaborative Robots: AI-powered robots can work alongside human workers, adapting to changes in their environment and performing complex tasks.
- Autonomous Mobile Robots: ML algorithms enable robots to navigate factory floors autonomously, optimizing material movement and logistics.
- Adaptive Manufacturing: AI allows robots to learn and adapt to new tasks, increasing flexibility in production processes.
6. Digital Twin Technology
Digital twin technology, powered by AI and ML, is providing manufacturers with virtual replicas of their physical assets and processes.
- Real-Time Monitoring: Digital twins can provide real-time insights into the performance of physical assets.
- Scenario Testing: Manufacturers can use digital twins to test different scenarios and optimize processes without disrupting physical operations.
- Predictive Analysis: ML algorithms can analyze digital twin data to predict future performance and potential issues.
7. Generative Design
AI-powered generative design tools are revolutionizing product development in manufacturing.
- Design Optimization: AI can generate multiple design options based on specified parameters, optimizing for factors like weight, strength, and cost.
- Rapid Prototyping: Generative design coupled with 3D printing allows for faster and more efficient prototyping.
- Material Optimization: AI can suggest optimal materials for specific design requirements, improving product performance and reducing costs.
8. Human-Machine Interaction
AI is enhancing the way humans interact with machines in manufacturing environments, improving safety and efficiency.
- Natural Language Processing: AI-powered interfaces allow workers to interact with machines using natural language commands.
- Augmented Reality: AI-enhanced AR systems can provide workers with real-time information and guidance during complex tasks.
- Gesture Recognition: Advanced AI algorithms can interpret human gestures, allowing for more intuitive control of manufacturing equipment.
9. Demand-Driven Production
AI and ML enable manufacturers to implement more agile, demand-driven production models.
- Real-Time Market Analysis: ML algorithms can analyze market data in real-time, allowing manufacturers to quickly adjust production to meet changing demand.
- Personalized Production: AI enables mass customization, allowing manufacturers to efficiently produce personalized products.
- Just-in-Time Manufacturing: ML can optimize production schedules to align closely with demand, reducing inventory costs.
10. Sustainability and Environmental Impact
AI and ML are playing a crucial role in helping manufacturers reduce their environmental impact and improve sustainability.
- Waste Reduction: ML algorithms can optimize processes to minimize waste generation.
- Energy Management: AI systems can manage energy consumption more efficiently, reducing carbon footprint.
- Sustainable Material Selection: AI can help identify and select more sustainable materials for manufacturing processes.
Aspect of Manufacturing Efficiency | AI/ML Application | Benefits |
Predictive Maintenance | Sensor data analysis, maintenance scheduling | Reduced downtime, lower maintenance costs |
Quality Control | Image recognition, real-time monitoring | Improved product quality, reduced defects |
Supply Chain Optimization | Demand forecasting, inventory optimization | Reduced costs, improved supply chain resilience |
Process Optimization | Production line analysis, energy efficiency | Increased productivity, reduced waste |
Robotic Process Automation | Collaborative robots, adaptive manufacturing | Enhanced flexibility, improved safety |
Digital Twin Technology | Real-time monitoring, scenario testing | Improved decision-making, reduced risks |
Generative Design | Design optimization, rapid prototyping | Faster product development, improved product performance |
Human-Machine Interaction | Natural language processing, augmented reality | Enhanced worker productivity, improved safety |
Demand-Driven Production | Real-time market analysis, personalized production | Improved responsiveness to market changes, reduced inventory costs |
Sustainability | Waste reduction, energy management | Reduced environmental impact, improved sustainability |
The impact of AI and ML on manufacturing efficiency has been profound, offering manufacturers powerful tools to optimize their operations, improve product quality, and reduce costs. As these technologies continue to evolve, we can expect even more innovative applications that will further transform the manufacturing landscape, driving increased productivity and sustainability.
C. Financial Services Transformation
The financial services industry has been at the forefront of adopting artificial intelligence and machine learning technologies. These advanced systems are revolutionizing everything from risk assessment to customer service, creating more efficient, secure, and personalized financial experiences. Let’s explore the various ways AI and ML are transforming financial services.
1. Fraud Detection and Prevention
AI and ML have significantly enhanced the ability of financial institutions to detect and prevent fraudulent activities.
- Real-Time Transaction Monitoring: ML algorithms can analyze transactions in real-time, flagging suspicious activities for immediate review.
- Anomaly Detection: AI systems can identify unusual patterns in transaction data that may indicate fraudulent activity.
- Behavioral Biometrics: ML can analyze user behavior patterns to detect potential account takeovers or identity theft.
2. Credit Scoring and Risk Assessment
AI-powered systems are revolutionizing credit scoring and risk assessment processes, making them more accurate and inclusive.
- Alternative Data Analysis: ML algorithms can analyze non-traditional data sources to assess creditworthiness, potentially expanding access to credit for underserved populations.
- Real-Time Risk Adjustment: AI can continuously update risk assessments based on new data, allowing for more dynamic and accurate risk management.
- Predictive Modeling: ML models can predict future financial behavior, helping institutions make more informed lending decisions.
3. Algorithmic Trading
AI and ML have transformed trading strategies, enabling more sophisticated and data-driven decision-making.
- High-Frequency Trading: ML algorithms can analyze market data and execute trades in milliseconds, capitalizing on small price movements.
- Sentiment Analysis: AI can analyze news and social media to gauge market sentiment and inform trading decisions.
- Pattern Recognition: ML can identify complex patterns in market data that human traders might miss.
4. Personalized Banking
AI is enabling banks to offer more personalized services and products to their customers.
- Customized Product Recommendations: ML algorithms can analyze customer data to suggest relevant financial products and services.
- Personalized Financial Advice: AI-powered robo-advisors can provide tailored investment advice based on individual financial goals and risk tolerance.
- Dynamic User Interfaces: AI can customize banking interfaces based on individual user preferences and behavior.
5. Customer Service and Chatbots
AI-powered chatbots and virtual assistants are transforming customer service in the financial sector.
- 24/7 Support: AI chatbots can provide round-the-clock customer support, answering queries and resolving issues.
- Natural Language Processing: Advanced NLP allows chatbots to understand and respond to customer queries in a more human-like manner.
- Sentiment Analysis: AI can analyze customer sentiment during interactions, allowing for more empathetic and effective responses.
6. Anti-Money Laundering (AML) and Know Your Customer (KYC)
AI and ML are enhancing AML and KYC processes, making them more efficient and effective.
- Pattern Recognition: ML algorithms can identify complex patterns indicative of money laundering activities.
- Document Verification: AI-powered systems can automate the verification of identity documents, speeding up the KYC process.
- Ongoing Monitoring: ML can continuously monitor customer activities for suspicious behavior, enhancing AML efforts.
7. Process Automation
AI and ML are automating many back-office processes in financial institutions, improving efficiency and reducing errors.
- Automated Underwriting: ML algorithms can automate much of the loan underwriting process, speeding up approvals.
- Claims Processing: AI can automate insurance claims processing, reducing processing times and improving accuracy.
- Regulatory Compliance: AI systems can help ensure compliance with complex financial regulations by automating reporting and monitoring processes.
8. Market Analysis and Forecasting
AI and ML are providing more accurate and timely market insights and forecasts.
- Predictive Analytics: ML models can analyze vast amounts of market data to predict future trends and market movements.
- Natural Language Processing: AI can analyze news articles, social media, and other text-based sources to gauge market sentiment.
- Alternative Data Analysis: ML can incorporate non-traditional data sources into market analysis, providing unique insights.
9. Asset and Wealth Management
AI is transforming asset and wealth management, offering more sophisticated and personalized investment strategies.
- Portfolio Optimization: ML algorithms can optimize investment portfolios based on individual risk profiles and market conditions
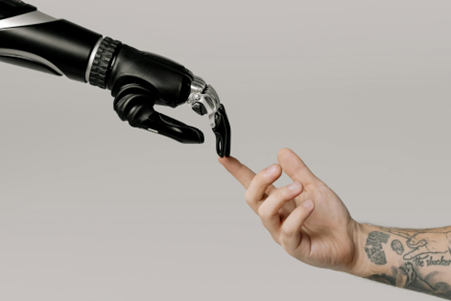
Portfolio Optimization
Ethical Considerations in AI and ML
As we delve deeper into the world of artificial intelligence and machine learning, it’s crucial to address the ethical implications that come with these revolutionary technologies. While AI and ML offer tremendous potential for innovation and progress, they also raise significant concerns that need to be carefully considered and addressed.
A. Job Displacement
One of the most pressing ethical concerns surrounding AI and machine learning is the potential for widespread job displacement. As these technologies continue to advance, they are increasingly capable of performing tasks that were once exclusively done by humans. This shift has far-reaching implications for the global workforce and economy.
The Scale of Potential Job Loss
The impact of AI and ML on employment is expected to be substantial. According to a study by the McKinsey Global Institute, up to 800 million jobs worldwide could be automated by 2030. This represents approximately 30% of the global workforce. While new jobs will undoubtedly be created as a result of these technologies, the transition period could be challenging for many workers.
Industries at Risk
Some industries are more vulnerable to AI-driven automation than others. Here’s a breakdown of sectors that are likely to see significant job displacement:
- Manufacturing: Robots and AI-powered systems are increasingly capable of performing complex assembly tasks.
- Transportation: Self-driving vehicles threaten jobs in trucking, taxi services, and delivery.
- Customer service: AI chatbots and virtual assistants are replacing human customer service representatives.
- Finance: Algorithmic trading and AI-powered financial analysis are reducing the need for human traders and analysts.
- Retail: Automated checkout systems and e-commerce are reducing the need for in-store staff.
The Human Cost
Job displacement due to AI and ML goes beyond mere statistics. It has profound implications for individuals and communities:
- Loss of income and financial stability
- Reduced sense of purpose and self-worth
- Increased inequality as high-skill jobs become more valuable
- Potential social unrest due to widespread unemployment
Mitigating Job Displacement
To address this ethical challenge, several strategies have been proposed:
- Retraining and upskilling programs: Governments and businesses should invest in programs to help workers transition to new roles that are less likely to be automated.
- Universal Basic Income (UBI): Some proponents argue for a UBI to provide financial security in the face of job uncertainty.
- Job creation in AI-related fields: As AI and ML grow, new jobs in development, maintenance, and oversight of these technologies will emerge.
- Collaborative AI: Developing AI systems that augment human capabilities rather than replace them entirely.
- Ethical AI development: Encouraging the development of AI that prioritizes human well-being and job preservation.
The Role of Policy and Regulation
Policymakers have a crucial role to play in addressing job displacement:
- Implementing education reforms to prepare future generations for an AI-driven job market
- Creating incentives for businesses to retrain workers rather than replace them
- Developing safety nets for workers displaced by automation
- Encouraging responsible AI development that considers societal impact
B. Privacy Concerns
As AI and machine learning algorithms become more sophisticated and pervasive, they raise significant privacy concerns. These technologies rely on vast amounts of data to function effectively, often including personal information about individuals.
Data Collection and Use
AI and ML systems collect and process enormous amounts of data, including:
- Personal information (name, age, address)
- Behavioral data (browsing history, purchase patterns)
- Biometric data (facial recognition, voice patterns)
- Location data
- Social media activity
This data is used to train algorithms, personalize experiences, and make predictions about user behavior. However, the extent of this data collection and its potential uses raise serious privacy concerns.
Potential Privacy Violations
The use of AI and ML can lead to various privacy violations:
- Unauthorized data access: Breaches or misuse of collected data can expose sensitive personal information.
- Invasive profiling: AI algorithms can create detailed profiles of individuals, potentially revealing more than they intend to share.
- Surveillance: AI-powered facial recognition and other technologies can enable pervasive surveillance.
- Data exploitation: Personal data may be used for purposes beyond what individuals consented to.
- Loss of anonymity: Advanced data analysis techniques can de-anonymize supposedly anonymous data.
The Right to Privacy in the Age of AI
The widespread use of AI and ML challenges traditional notions of privacy:
- Informed consent: It’s increasingly difficult for individuals to understand how their data is being used and to give meaningful consent.
- Data ownership: Questions arise about who owns the data generated by AI systems and how it can be used.
- Right to be forgotten: The persistence of data in AI systems makes it challenging to fully delete personal information.
Regulatory Responses
Several regulatory frameworks have been developed to address AI-related privacy concerns:
- General Data Protection Regulation (GDPR): This EU regulation sets strict rules for data protection and privacy.
- California Consumer Privacy Act (CCPA): Similar to GDPR, this law gives California residents more control over their personal data.
- AI-specific regulations: Some jurisdictions are developing AI-specific privacy laws to address unique challenges posed by these technologies.
Ethical AI Development for Privacy Protection
To address privacy concerns, AI developers and companies should adopt ethical practices:
- Privacy by Design: Incorporating privacy protections into AI systems from the outset.
- Data minimization: Collecting and retaining only the data necessary for the intended purpose.
- Transparency: Clearly communicating how data is collected, used, and protected.
- User control: Providing individuals with options to control their data and opt out of data collection.
- Robust security measures: Implementing strong safeguards to protect collected data.
The Balance Between Innovation and Privacy
Finding the right balance between leveraging AI for innovation and protecting individual privacy is a complex challenge. It requires ongoing dialogue between technologists, policymakers, ethicists, and the public to develop solutions that respect privacy while allowing for technological advancement.
C. Bias in Algorithms
As AI and machine learning systems become increasingly influential in decision-making processes across various domains, the issue of algorithmic bias has emerged as a critical ethical concern. Bias in AI algorithms can lead to unfair or discriminatory outcomes, perpetuating and even amplifying existing societal inequalities.
Understanding Algorithmic Bias
Algorithmic bias refers to systematic and repeatable errors in a computer system that create unfair outcomes, such as privileging one arbitrary group of users over others. In the context of AI and ML, bias can manifest in various ways:
- Training data bias: When the data used to train an AI model is not representative of the population it will be applied to.
- Algorithm design bias: When the structure or logic of an algorithm inherently favors certain outcomes.
- Interaction bias: When the way users interact with an AI system influences its behavior in biased ways.
- Deployment bias: When an AI system is applied in contexts or to populations different from those it was designed for.
Examples of AI Bias
To understand the real-world implications of algorithmic bias, consider these examples:
- Facial recognition systems: Many facial recognition algorithms have been found to perform less accurately on people of color and women, leading to potential misidentifications.
- Hiring algorithms: AI-powered recruiting tools have shown bias against women and minorities, perpetuating workplace discrimination.
- Criminal justice risk assessment: Algorithms used to predict recidivism rates have been found to incorrectly label black defendants as higher risk compared to white defendants.
- Credit scoring: AI-driven credit scoring systems may unfairly deny loans to individuals from certain demographic groups.
- Healthcare algorithms: Some healthcare AI systems have been found to allocate less care to black patients than to equally sick white patients.
The Impact of Algorithmic Bias
The consequences of bias in AI and ML algorithms can be far-reaching and severe:
- Reinforcing societal inequalities: Biased algorithms can perpetuate and amplify existing social, economic, and racial disparities.
- Unfair decision-making: In critical areas like healthcare, criminal justice, and finance, biased algorithms can lead to unjust outcomes for individuals.
- Erosion of trust: As instances of algorithmic bias come to light, public trust in AI systems and the institutions using them may decrease.
- Legal and regulatory risks: Companies using biased AI systems may face legal challenges and regulatory scrutiny.
Addressing Algorithmic Bias
Mitigating bias in AI and ML systems is a complex challenge that requires a multi-faceted approach:
- Diverse and representative data: Ensuring training data is diverse and representative of the population the algorithm will serve.
- Algorithmic fairness techniques: Implementing mathematical techniques to detect and mitigate bias in algorithms.
- Regular auditing: Continuously monitoring AI systems for bias and unfair outcomes.
- Diverse development teams: Promoting diversity in AI development teams to bring varied perspectives to algorithm design.
- Transparency and explainability: Making AI decision-making processes more transparent and interpretable.
- Ethical guidelines: Developing and adhering to ethical guidelines for AI development and deployment.
The Role of Regulation in Combating Algorithmic Bias
Regulatory bodies are beginning to address the issue of algorithmic bias:
- The EU’s proposed AI Act includes provisions for high-risk AI systems to be assessed for bias before deployment.
- The US Federal Trade Commission has issued guidance on using AI and algorithms, emphasizing the importance of fairness and non-discrimination.
Ethical Frameworks for AI Development
Several ethical frameworks have been proposed to guide the development of fair and unbiased AI systems:
OECD AI Principles: Promote AI that is innovative, trustworthy, and respects human rights and democratic values.
IEEE Global Initiative on Ethics of Autonomous and Intelligent Systems: Provides guidelines for ethically aligned design of AI systems.
AI Ethics Guidelines for Trustworthy AI: Developed by the European Commission, emphasizing human agency, fairness, and transparency.
The Intersection of Bias and Other Ethical Concerns
Algorithmic bias intersects with other ethical considerations in AI:
Ethical Concern | Intersection with Bias |
Privacy | Biased algorithms may disproportionately invade the privacy of certain groups |
Transparency | Lack of transparency can make it difficult to identify and address bias |
Accountability | Determining responsibility for biased outcomes in complex AI systems |
Human Rights | Biased AI can infringe on fundamental human rights and dignities |
The Ongoing Challenge of Fairness in AI
Addressing bias in AI and ML algorithms is an ongoing process that requires constant vigilance and adaptation. As AI systems become more complex and are applied to new domains, new forms of bias may emerge. The tech industry, academia, and regulatory bodies must work together to develop robust strategies for identifying, mitigating, and preventing algorithmic bias.
As we look to the future, several trends are emerging in the fight against algorithmic bias:
- AI fairness tools: Development of open-source tools and frameworks for detecting and mitigating bias in AI systems.
- Interdisciplinary approach: Increased collaboration between computer scientists, ethicists, social scientists, and legal experts to address bias.
- Regulatory frameworks: Evolution of more comprehensive and nuanced regulations specifically addressing AI bias.
- Education and awareness: Greater emphasis on educating developers, users, and the public about algorithmic bias and its implications.
- Bias-aware AI: Development of AI systems that are inherently designed to detect and correct their own biases.
In conclusion, addressing ethical considerations in AI and ML, particularly job displacement, privacy concerns, and algorithmic bias, is crucial for ensuring that these powerful technologies benefit society as a whole. As we continue to navigate these challenges, it’s essential to maintain a balance between technological progress and ethical responsibility. By doing so, we can harness the full potential of AI and ML while safeguarding fundamental human rights and values.
As we move forward, it’s important to consider how these ethical considerations will shape the future development and application of AI and ML technologies. The next section will explore emerging trends and potential future directions in the field of artificial intelligence and machine learning.